
Ongoing Research Themes
High-Dimensional Statistical Learning
High-dimensional data, in which the number of features exceeds the number of observations, results in both theoretical and methodological challenges. We develop approaches to overcome these challenges by exploiting structure in the data or in the underlying model. We are particularly interested in unsupervised learning, with a focus on graphical modeling.
Statistical Models for Neural Activity
Recent technological advances have made it possible to simultaneously record from huge numbers of neurons. This leads to a number of questions:
what is the functional connectivity among a population of neurons?
can we identify functional sub-populations of neurons?
how can we model a neuron’s activity as a function of covariates?
We are pursuing answers to these questions, and others, in collaboration with colleagues at the Allen Institute for Brain Science and Princeton University.
“Double-DiPPING” AND SELECTIVE Inference
As the scope and scale of data collection continue to increase, researchers are increasingly collecting data in order to “find something interesting” — in other words, to generate a hypothesis. Unfortunately, testing this hypothesis on the same data leads to a “double-dipping” problem: classical statistical approaches require a hypothesis to be specified in advance, not generated from the same data used for testing. From a statistical perspective, double-dipping results in uncontrolled selective Type 1 error. We are developing a selective inference framework that can be used to test data-generated hypotheses for a number of very popular methods for data analysis, including hierarchical clustering, regression trees, and more.
Multi-View Data
It is becoming increasingly common for researchers to collect multiple data views — that is, sets of features — on a single set of observations. For instance, researchers might collect clinical as well as gene expression measurements for a single set of patients. We are developing approaches to exploit the availability of multiple data views in order to answer questions that could not be answered if each data view were collected on a separate set of observations.
Applications
Our work is motivated by diverse applications both in and out of the biomedical sciences. It has recently been inspired by collaborations with researchers in genomics, neuroscience, microbial ecology, and pathology. We are always open to new and interesting collaborations!
Featured Publications

Recent News
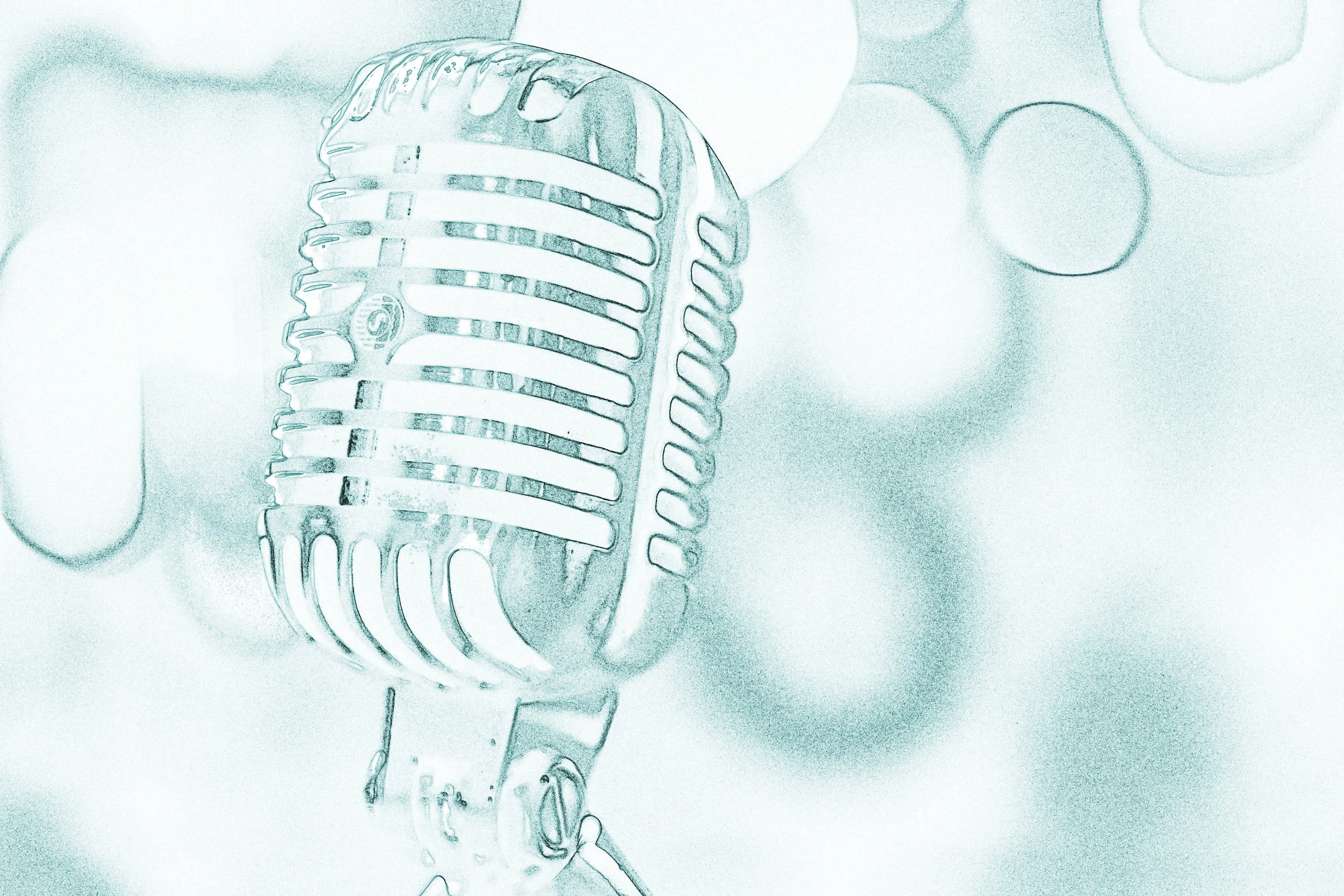